RELand: Risk Estimation of Landmines via Interpretable Invariant Risk Minimization
Mateo Dulce Rubio*, Siqi Zeng*, Qi Wang, Didier Alvarado (UNMAS), Francisco Moreno (CCCM), Hoda Heidari, Fei Fang
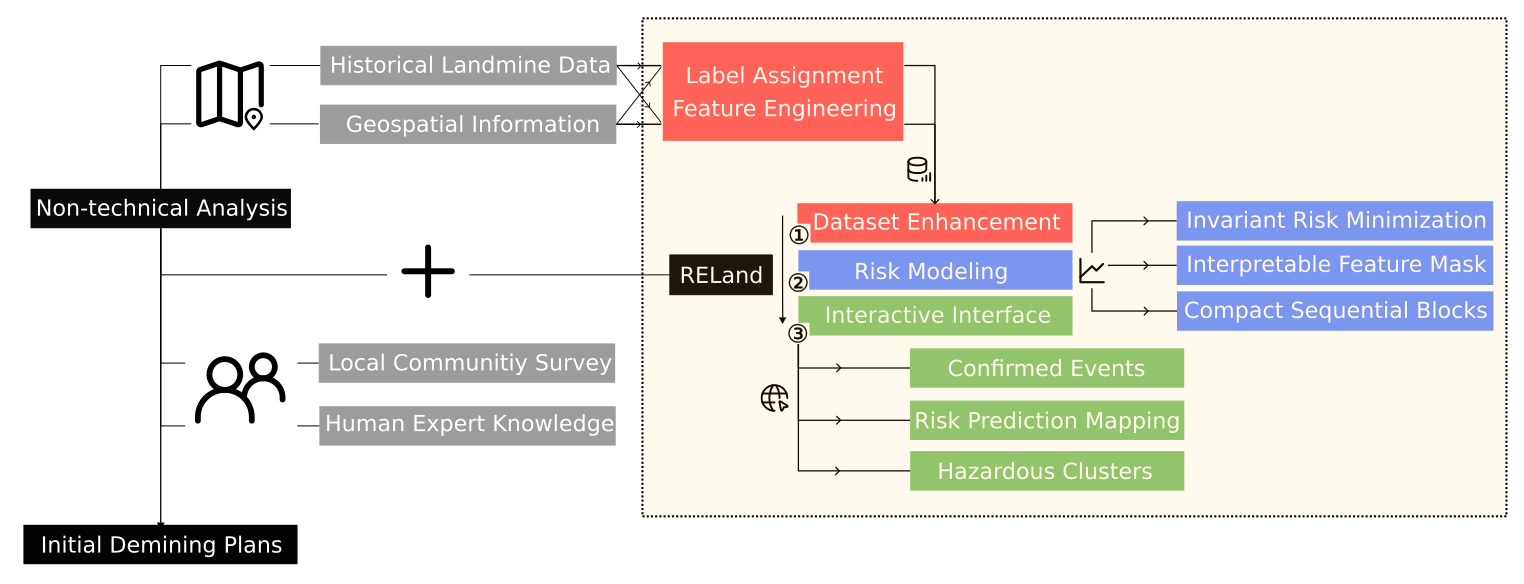
Abstract
Landmines remain a threat to war-affected communities for years after conflicts have ended, partly due to the laborious nature of demining tasks. Humanitarian demining operations begin by collecting relevant information from the sites to be cleared, which is then analyzed by human experts to determine the potential risk of remaining landmines. In this paper, we propose RELand system to support these tasks, which consists of three major components. We (1) provide general feature engineering and label assigning guidelines to enhance datasets for landmine risk modeling, which are widely applicable to global demining routines, (2) formulate landmine presence as a classification problem and design a novel interpretable model based on sparse feature masking and invariant risk minimization, and run extensive evaluation under proper protocols that resemble real-world demining operations to show a significant improvement over the state-of-the-art, and (3) build an interactive web interface to suggest priority areas for demining organizations. We are currently collaborating with a humanitarian demining NGO in Colombia that is using our system as part of their field operations in two areas recently prioritized for demining.
Reference
RELand: Risk Estimation of Landmines via Interpretable Invariant Risk Minimization
Mateo Dulce Rubio*,
Siqi Zeng*,
Qi Wang,
Didier Alvarado (UNMAS),
Francisco Moreno (CCCM),
Hoda Heidari,
Fei Fang
ACM Journal on Computing and Sustainable Societies 2 (2) (ACM JCSS). 2024.
PDF
Presentación en español
* Authors contributed equally